Introduction
The ocean covers more than 70% of the earth, harbouring diverse marine biodiversity, including seaweeds (algae). Algae are known for their photosynthesizing ability, ranging from Cyanobacteria to complex giant kelps. These macroscopic marine algae are treasured for ecological significance in the aquatic ecosystem and their utilization in food, cosmetics, and other industries, thereby considered as a potential and unexplored resource for discovering drugs (Castro, Cotas, Gomes, Pacheco, & Pereira, 2023; Kannan R.R. Rengasamy et al., 2014; Kumari, Garima, & Bharadvaja, 2023; Pereira & Valado, 2023). These seaweeds are packed with bioactive products with the properties to fight against cholesterol, bacteria, inflammation, oxidation, allergies, itchiness, fungus, cancer, Lung damage, immune alteration, and neural and chemical damage. Helpful in developing novel food and nutraceuticals to prevent physiological issues like cancer, diabetes, cardiovascular disease, arthritis, autoimmune disease, and ocular diseases (Alves et al., 2018; Cotas et al., 2021; Leandro et al., 2020; Lopes, Sousa, Valentão, & Andrade, 2013; Tanna & Mishra, 2018). Phaeophyceae is a diverse brown algae rich in fucoxanthin and pheophytin tannins, giving it a characteristic greenish-brown colour. These algae are packed with secondary metabolites like phlorotannin. About 1140 metabolites are registered, of which brown algae contains huge polysaccharides like alginate and fucoidans, known for their ability to mitigate proliferation, cancer, inflammation, cholesterol and viral activities (Chakraborty, Joseph, & Praveen, 1924; Cox, Gupta, & Abu-Ghannam, 2012; Hakim & Patel, 2020; Kolanjinathan, Ganesh, & Saranraj, 2014). Additionally, brown algae aids in conditions like asthma, haemorrhoids, hypothyroidism, cough, headaches, stomach pain, weight loss, and skin problems by reducing inflammation and thinning the blood (Cumashi et al., 2007; Dürig et al., 1997; Hakim & Patel, 2020). Brown algae are more effective thaned and green due to higher antioxidant levels and phenolic compounds (Mekinić et al., 2019).
Lessoniaceae family edible Kelp (brown algae), Ecklonia genus comprises nine species Ecklonia biruncinata, Ecklonia brevipes, Ecklonia cava (EC), Ecklonia kurome (EK), Ecklonia muratii, Ecklonia radiata (ER), Ecklonia fastigiata, Ecklonia maxima (EM), and Ecklonia stolonifera (ES) (Hornemann, 1828; Moon et al., 2008) primarily rich in eckol variant phlorotannins and used as traditional medicine plus food in countries of East-Asia like Korea, China, and Japan (Koirala, Jung, & Choi, 2017). This seaweed is nutrient-dense with many phytochemicals like fucoidans (polysaccharides with Sulphur), carotenoids, peptides, and phlorotannins helpful in providing therapeutic properties (Cho et al., 2012; Gunathilake, Taiwo, Wang, & Colin, 2024; Kang et al., 2013; Lee & Jeon, 2013; Montero et al., 2016; Priyanka, Rajaram, & Sivakumar, 2022; Shin, Hwang, Kang, & Lee, 2006).
Novel drug development is vital due to varied challenges occurring due to diseases like neurodegenerative, infections, or cancer and the constraints of the existing therapies. Seaweeds are largely unexplored natural product sources, with the ability to outlive extreme marine conditions and possibility to act as a promising agent for the pharmaceutical industry (Bhatia et al., 2022; Makhoba, Viegas, Mosa, Viegas, & Pooe, 2020; Pereira & Valado, 2023).
The main motive of our study is to delineate the bioactive components of the Ecklonia species using pharmacological network method to identify potential therapeutic targets plus identifying the interaction site of compounds with the significant genes associated to the pathogenesis of Alzheimer's (AD), coronary artery disease (CAD) and type-2 diabetes (T2D) diseases. Immune activation properties are shown by the protease extracts obtained from Ecklonia cava by activating NF-kB pathways and lymphocyte via IL-2 release (Kuznetsova, Persiyanova, Ermakova, Khotimchenko, & Besednova, 2018). Inspired by study of Fucoxanthin anti-bacterial property obtained from stolonifera towards Gram-positive bacteria like Staphylococcus aureus, Streptococcus agalactiae, Staphylococcus epidermidis, Serratia marcescens, Pseudomonas aeruginosa, Proteus mirabilis (Menaa et al., 2021).
Materials and Methods
Network pharmacology analysis was performed on the compounds targeting AD, CAD and T2D in the context of the research. The structures of the compounds obtained from Ecklonia were extracted from MarinLit, a database dedicated to marine natural products SMILES (Kim et al., 2023). The Swiss Target Prediction tool (Daina, Michielin, & Zoete, 2019) predicted the potential targets, and 0.1 is the minimum probability score for screening. The Open Targets Platform (Ochoa et al., 2023) illustrated the potential therapeutic targets for AD (ID: MONDO_0004975), CAD (ID: EFO_0001645), and T2D (ID: MONDO_0005148), with further refining of the overall association score to 0.3 or more. The BioTools.fr tool was used to generate Venn diagrams to identify the common targets, followed by the compound-target network visualization through Cytoscape_v3.10.2 in a circular and grid configuration (Shannon et al., 2003). The STRING database (Szklarczyk et al., 2019) allowed us to detect protein-protein interaction (PPI) networks by analysing the frequent target between Ekcolina's compounds and the diseases, with a threshold confidence greater than 0.4 for minimal interaction. These networks were imported into Cytoscape software for investigation. The Hub genes were identified in the PPI network using the tool Cytohubba of the Cytoscape. The connections between the PPI network nodes were represented as data strength based on their confidence level and edge thickness. Followed by the detection of common targets inputted into ShinyGO 0.77 (Ge, Jung, Jung, & Yao, 2020). According to 36 and 37, our data was subjected to gene ontology prediction and KEGG metabolic pathway analysis. The study obtained the top 20 gene ontologies associated with biological processes, components of cells, functions of molecules and KEGG routes. Bar plots represented the number of genes related to each function and pathway.
Later, we developed a network showcasing the critical KEGG pathways by calculating the gene overlapping percentage to further provide critical insights into metabolic pathways. Finally, the typical targets' genomic coordinates were retrieved and displayed on a genome plot for analysis. In addition, Swiss ADME, a technique created by 31, is utilized to assess the physicochemical and pharmacokinetic properties of the molecule. The compounds were fed canonically in SMILES representation to yield valuable information about several physicochemical properties, like the characteristics of hydrogen bonds, the refractive index of the molecule, and solubility in lipids and water. Moreover, it explored several pharmacological kinetic characteristics such as absorption in the gastrointestinal tract, inhibition of cytochrome P450 enzymes, therapeutic properties, and significant issues in medicinal chemistry (given as supplementary).
Results and Discussion
Utilizing Swiss Target Prediction analysis, we concluded the identified potent targets are linked to 16 clear-cut compounds. Predictions were filtered according to the probability score threshold from 0.10 or higher were ensured as a comprehensive scope. This deliberate selection criterion encompassed different probable targets specific to the investigated compounds. We revealed 297 unique target points linked with the 16 bioactive compounds. Additionally, leveraging data from the Open Target Platform focused on AD, T2D, and CAD, we identified 4611, 7627, and 4402 targets, of 56,124 and 167 probable targets were filtered according to their overall association score <0.50 to prioritize the robust association with the disease targets. The overall association score was calculated by integrating factors like genetics, somatic alterations, therapeutics targets, pathways, systemic biology, data mining, RNA expression, and animal model investigations.
Figure 1
Venn diagram depicting the overlap of compounds associated with coronary artery disease, type 2 diabetes, and Alzheimer's disease.
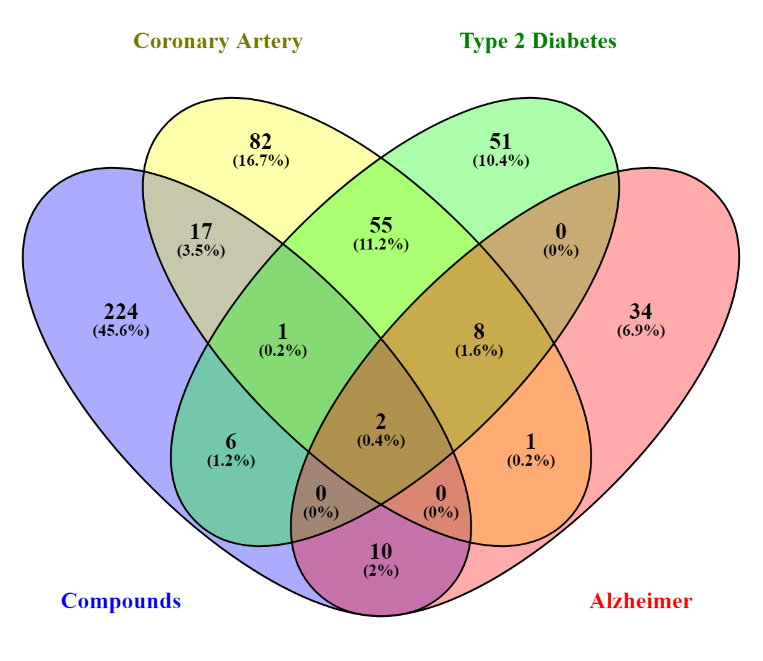
Figure 2
Network diagram illustrating the interactions between plant compounds and their molecular targets across three diseases: coronary artery disease, type 2 diabetes, and Alzheimer's disease.
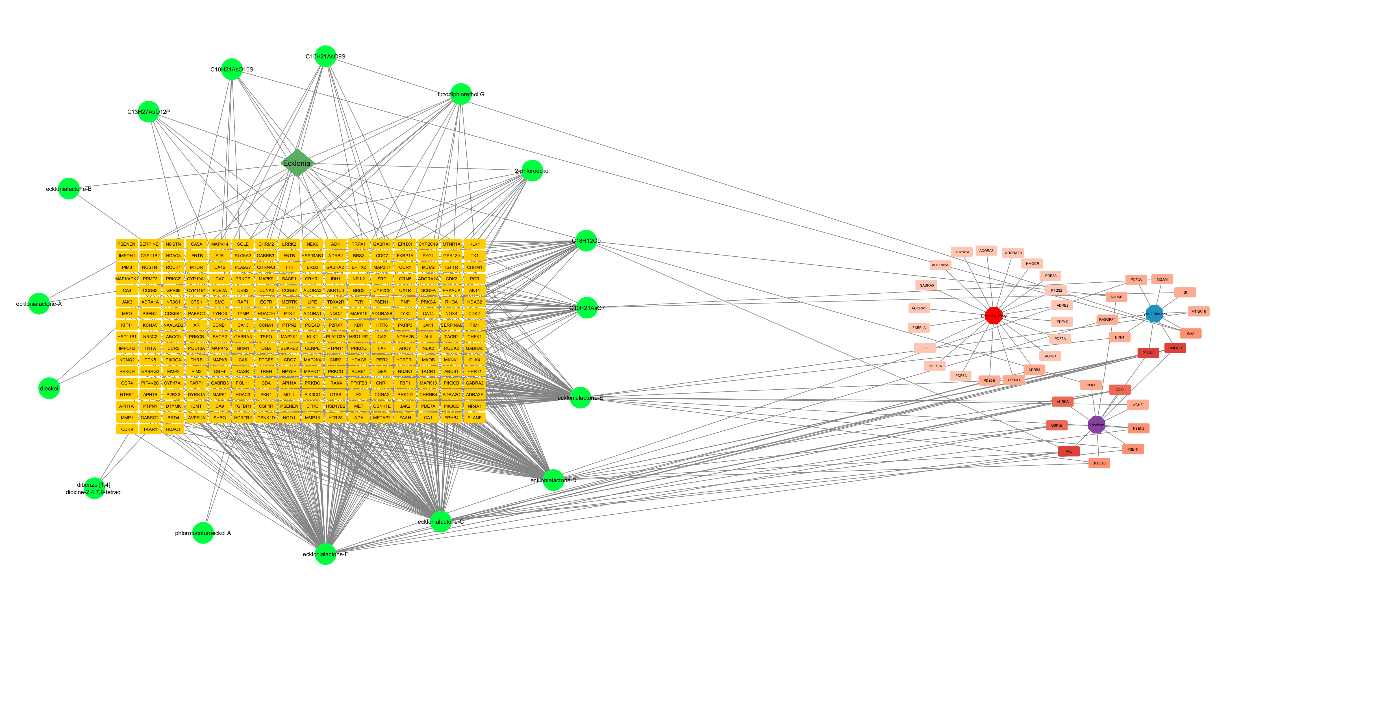
Venn Diagram analysis revealed two mutual targets between Ekcolinia's compound and the three diseases (Figure 1). Previous studies showed polyphenols from the marine algae are linked to higher antioxidant levels specially the phlorotannins, eckol, triphlorethol A are nruroprotective, anti-inflammatory rich (Lomartire & Gonçalves, 2023; Zheng, Zhao, & Guo, 2022). Subsequent visualization using a circular network format with Cytospace was conducted to find the interaction between the compound targets. The green nodes denote the Ekcolina compound, as the yellow nodes correlated with the compound's predicted targets. Red nodes indicate the common targets of the disease and Ekcolinia's compounds (Figure 2). Statistical analysis of the properties showed a compound-target network with a well-connected topology comprising 318 nodes and 592 edges, a six network diameter, an average number of neighbours of 3.723, and a characteristic path length of 3.226.
Figure 3
STRING interaction network displaying protein-protein interactions for Alzheimer's. (B) STRING interaction network displaying protein-protein interactions for Coronary Artery. (C) STRING interaction network displaying protein-protein interactions for Type 2 Diabetes. (D) STRING interaction network displaying protein-protein interactions for Type 2 Diabetes. (E) STRING interaction network displaying protein-protein interactions for Type 2 Diabetes. (F) STRING interaction network displaying protein-protein interactions for Type 2 Diabetes.
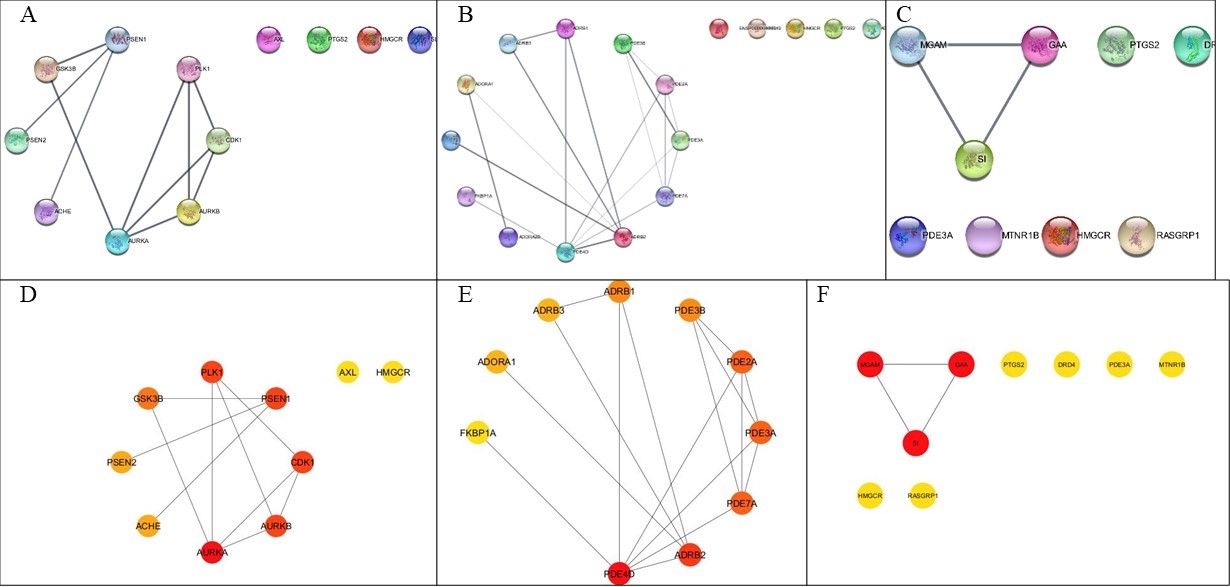
The interaction network of proteins constructed by the STRING database with a confidence threshold above 0.7 for AD, T2D, and CAD with 8, 3, and 10 connected targets, respectively, revealing the function and physical interactions between these proteins. Notably, the interactions between these proteins surpassed anticipated levels, emphasizing the significance of functional and biological interconnectedness (Figure 3). Table 1 shows further analysis of AD, T2D and CAD after importing the network separately into Cytoscape. We observed nodes and edges count, with an average neighbour per node, indicative of significant connectivity within the network. The network density represents the ratio of real and expected edges to exhibit a characteristic path length between them. The network diameter indicates the shortest distance between the most distant nodes, and the network displays a closed loop, suggesting a circular flow of interaction. Moreover, the node arrangement formed clusters, represented as clustering coefficients, indicating the presence of cohesive protein communities within the network.
Table 1
Statistical Observation of Protein-Protein Interaction Network:
The shared targets of AD, T2D and CAD were thoroughly investigated for their involvement in biological function, molecular functions, and pathway associations utilizing gene ontology and KEGG pathway analysis (Figure 4, Figure 5, Figure 6, Figure 7, Figure 8 ). The dot plot visually depicted the gene ontology, involvement in biological processes, functions at the molecular level and participation of each cell. The genes involved (Figure 9). To illustrate the location of the common genes on the chromosome, a bar graph is shown on the genome plot (supplementary). Analysing the pathway showcased the predominant involvement of Ekcolnia's compounds in the Alzheimer's pathway, Vascular Smooth Muscle Contraction pathway, Galactose Metabolism pathway, Starch and Sucrose Metabolism pathways for AD, T2D and CAD. Primary gene targets within the pathways include PSEN, GSK3B, ADRA1, ADORA2, PTGS2, and MGAM. Among the compounds analysed, Ekcolnialactone-E and Ekcolnialactone-C were predicted to interact with PSEN, and Ekcolnialactone-D, Ekcolnialactone-C, and Ekcolnialactone-F were found to act upon GSK3B. Additionally, Ekcolnialactone-F and Ekcolnialactone-D showed interactions with HMGCR and PTGS2. In the compound-target network analysis, HMGCR and PTGS2 displayed the the highest degree of in-degree between the common targets linked to all three diseases: AD, T2D anaysis. Our results were in association with the previous studies showing the modulation of pathways of calcium homeostasis, AMP-activated protein kinase (AMPK), cyclocxygenase, hyaluronidase and lipoxygenase (Kojima-Yuasa, 2018; Lu, Je, Hwang, Jeon, & Ryu, 2021; Sugiura, Katsuzaki, Imai, & Amano, 2021).
Figure 5
KEGG pathway analysis highlights the biological pathways enriched in Coronary artery disease.
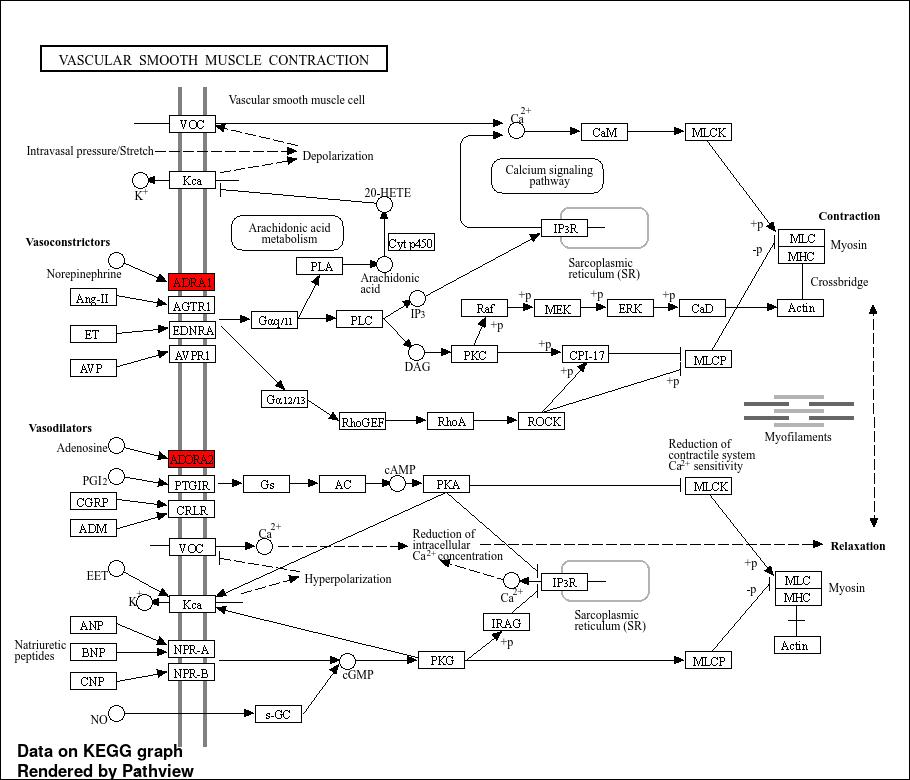
Figure 8
Bar plot representing Kegg Pathway Analysis and its associated disease: Alzheimer's, Coronary Artery and Type 2 Diabetes.
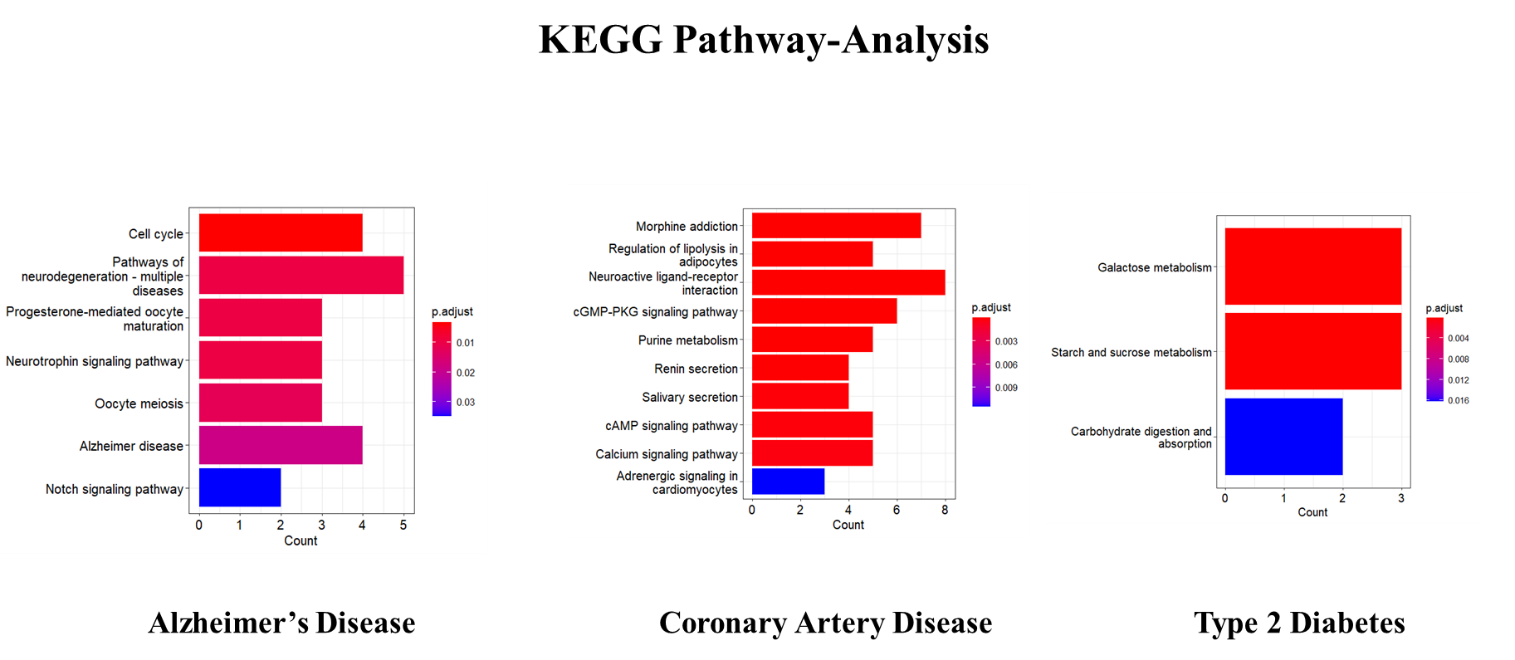
Figure 9
Dot plot illustrating the relationship between key variables (Biological Process, Cellular Component, and Molecular function) across Alzheimer's disease, coronary artery disease and Type 2 Diabetes.
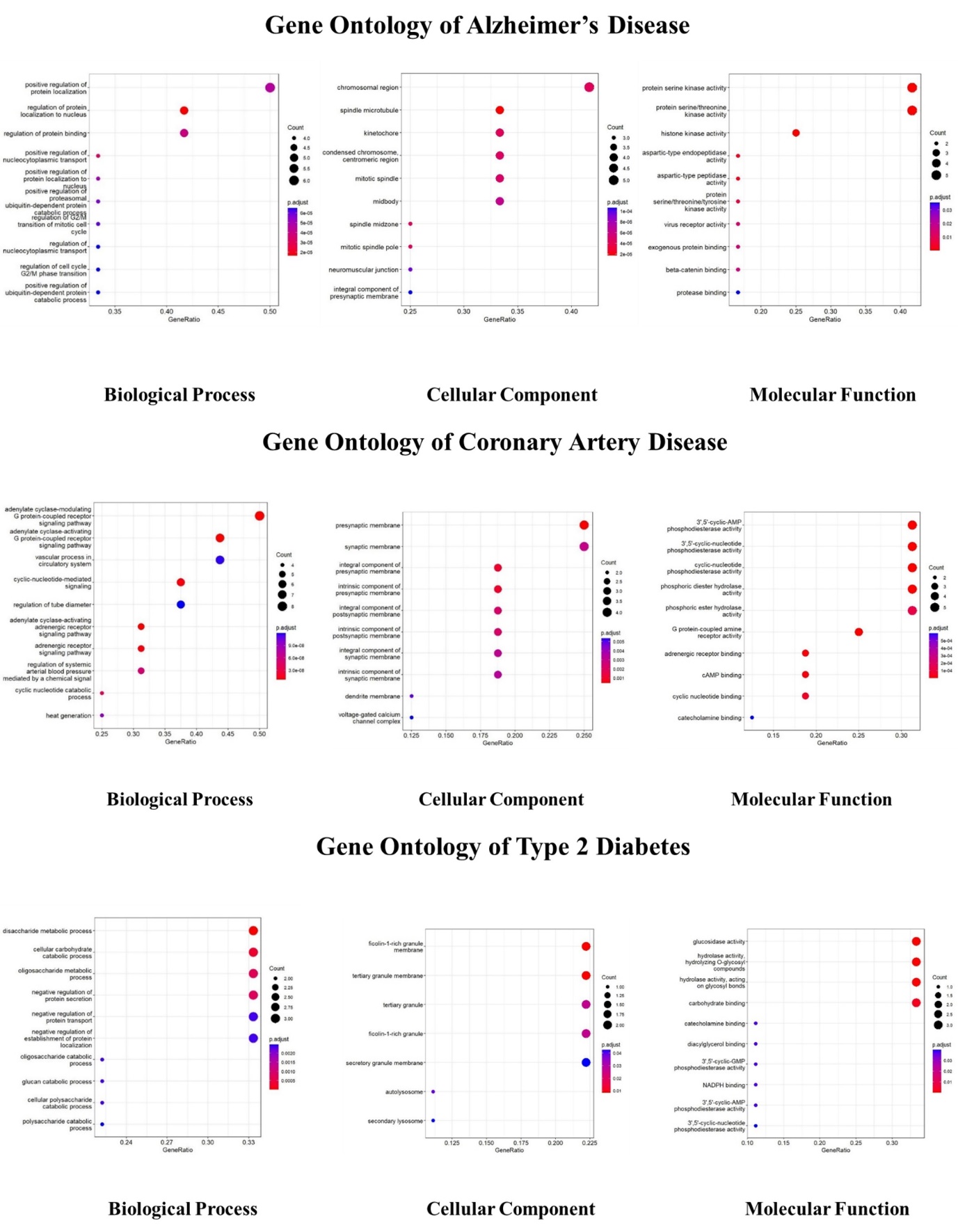
The HMGCR gene (GeneID: 3156) in humans is situated on chromosome 5q12 and encodes with three isoforms (1, 2, and 3) produced by alternative splicing. These isoforms' response varies with statin treatment (an HMGCR inhibitor). Isoform 1 of HMGCR has been extensively investigated due to about 888 amino acids in the glycoprotein membrane that regulates mevalonate, the initial checkpoint for endogenous cholesterol biosynthesis in the liver and small intestine. Varied mechanisms govern the amount and activity of HMGCR at multiple junctions, such as negative feedback regulatory mechanisms due to sterol and nonsterol metabolites from mevalonate, alteration during the post-translation process, breakdown, and hormone regulation. Cholesterol homeostasis is regulated by different regulatory pathways (Hermanto, Warsito, Rifa’i, & Widodo, 2023; Humphries et al., 1985). Ekcolina derivatives have shown potential inflammatory response modulation by interacting with the HMGCR gene. HMGCR variants are nearly identical to one another per unit drop in low-density lipoprotein (LDL) cholesterol level is linked with the risk of cardiovascular events and diabetes (Ference et al., 2016). According to research, low LDL levels have been linked to a lower risk of AD (Benn, Nordestgaard, Frikke-Schmidt, & Tybjærg-Hansen, 1648). Ekcolnia derivatives have been predicted to interact with the HMGCR gene, suggesting their potential to modulate the Alzheimer's pathway, Vascular Smooth Muscle Contraction pathway, Galactose Metabolism pathway, Starch and Sucrose Metabolism pathways. These results were in association with previously published work showing the influence of the compound in inhibiting the enzymes of HMGR (Coelho & Pacheco, 2023; Phan, Dayspring, & Toth, 2012). The in-silico evidence of Ekcolnia compounds in our study suggests ligand-receptor interactions. This finding supports Ekcolnia as a potent therapeutic tool for addressing AD, T2D, and CAD. Understanding Ekcolnia’s pharmacological properties of inhibiting HMGCR could pave the way for developing novel therapeutic interventions. Future investigation into these derivatives' specific modes of action and efficacy can further validate this super brown algae therapeutic potential.
Conclusion
Rising interest in natural products majorly obtained from marine seaweeds has drawn researchers towards brown algae because of their ability to synthesize a vast bundle of secondary bioactive compounds, which are considered better recent therapeutics. Our study highlighted the interacting system and the signature binding sight of the naturally obtained bioactive compound from Ecklonia species, brown edible algae. We saw favourable ligand-receptor binding with the genes HMGCR, PTGS2, ADRB2, AURKA, and PDE4D necessary for cholesterol synthesis, embryo development, bronchodilation, ventricular function, vasodilation, mitosis regulation, and breakdown of cAMP to cGMP. Our study suggests this species as a promising pharmaceutical herb for the drug development of diseases like AD, T2D and CAD. The chemical scaffolds of the Ecklonia can aid in the designing and developing modern anti-diabetic, anti-vasodilatory drugs with lesser side effects . These can be used as adjuvant to conventionally available therapeutics.
Competing Interests
Given his role as Associate Editor, Balamuralikrishnan Balasubramanian has not been involved and has no access to information regarding the peer review of this article. Full responsibility for the editorial process for this article was delegated to Associate Editor Shafi Ullah Khan. The authors declare no competing interests.
Acknowledgments
The authors acknowledge the financial support provided by the Department of Science and Technology for this research work.
Author contributions
This research article is done by collaborating with the authors. Conceptualization, S.V., H.K.; Writing original manuscript, S.V., H.K.B; Methodology, Data curation, Formal analysis, S.V., B.B., H.K.B.; Interpretation, Review and editing, B.B., H.K., F.M All the authors revised and approved the final article.