Introduction
Globally cardiovascular death from 1990 to 2019 was 18.56 million reported in the “Cause of Death” by Our World in Data website. In India, cardiovascular mortality led to 2.57 million deaths (Ritchie, 2018). Cardiovascular death (CVD) is associated with Heart attacks and strokes, which are acute events caused by the blockage of blood to the heart and brain, respectively (WHO, 2021). The blockage in the blood vessel is caused by the accumulation or build-up of fat molecules, cholesterol and other substances in the walls of large arteries and develops a sticky substance called plaque. The development of plaque and activation of cascading pathways like activation of inflammatory pathways and vessel narrowing was known as Atherosclerosis (Jebari-Benslaiman et al., 2022). Globally, these days the most frequent key cause of cardiovascular disease is “Atherosclerosis”. One of the best treatment strategies for atherosclerosis was reducing blood cholesterol levels (Ennezat et al., 2022). Many studies reported that few cholesterols lowering drugs were commercially available in the market (statins: HMG-CoA reductase inhibitor, Ezetimibe: NPC1L1 inhibitor, Alirocumab against PCSK9). But these treatments mainly involve a “one target-one drug” approach only. Various complex regulatory pathways were involved in atherosclerosis, associated with other cardiovascular risk factors like sex, smoking and mainly diabetes mellitus (Lechner et al., 2020). The ample evidence showed that type 2 diabetes mellitus was associated with atherosclerosis-related cardiovascular diseases (Ahmad et al., 2015). The low levels of HDL-cholesterol (High-density lipoprotein cholesterol) and elevated LDL-cholesterol (Low-density lipoprotein cholesterol) favour dyslipidaemia and increases the atherosclerotic-CVD risks in Type 2 diabetes mellitus patients (Duca, Sippl, & Snell-Bergeon, 2013). Likewise, the complexity associated with atherosclerotic CVD increases, and the current treatment strategy, “One drug, one target”, fails because complex diseases like atherosclerotic CVD, Diabetes Mellitus, and cancer were caused by the malfunction in the complete regulatory network rather than single gene mutation or dysfunction. So, the multi-target approach to treat complex diseases combines multiple drugs or single drugs for multiple targets (Ahmed, Samantasinghar, Soomro, Kim, & Choi, 2023). The treatment strategy for such complex diseases can be achieved by Network Pharmacology which is an In-silico approach of constructing an integrative network of “Compound/drug-gene-disease” to understand the synergistic therapeutic mechanism underlies the action of the drug or traditional medicine (Yuanyuan, Qiong, Hongxun, & Xu, 2023). The research in network pharmacology focuses on figuring out multiple target genes for drugs, compounds, and diseases, building protein-protein interaction (PPI) networks, and analysing networks for the therapeutic effect of drugs/compounds on multiple targets of complicated diseases like atherosclerosis and Diabetes Mellitus. Over the years, in the search for novel drug compounds for the “one drug, one target approach”, we forgot the traditional medicine using natural products from plant leaves, flowers, bark & seed (Ahmad et al., 2021; Kanu, John, Bahsoon, Kanu, & Kandeh, 2010). The natural compounds had the potential to bind to multiple target proteins and regulate various cellular targets associated with disease, making them play a significant role in medicine. Medicinal plants have numerous bioactive compounds that act upon multiple targets and elucidate cumulative activity. In ayurvedic (Indian Traditional medicine) and Chinese traditional medicine, the leaf, flower, rhizome and seed extract of Nelumbo nucifera, commonly referred to as scared lotus, which belongs to the Nelumbonaceae family was used to treat insomnia, hypertension, diarrhoea and cardiac ailments. Because of their medicinal value and rich nutritional contents (Chouaibi et al., 2012), the lotus was mainly cultivated in India, China, Japan and a few South East Asian & European countries (Guo, 2009). The lotus seeds or Nelumbinis semen were used as functional food, and it contains 61%-62% of carbohydrates, 16%-21% of total proteins, 2.40%-3% of crude fat and 5%-9% of moisture. The Resistant starch type 3 was the predominant carbohydrate in lotus seeds, enhancing gut microbiota growth and improving gastrointestinal health. The lotus seeds also contain various phytochemicals (Chen et al., 2012) like alkaloids, terpenoids and flavonoids. The bioactive compounds of lotus seeds extracts were reported to have anti-inflammatory, antidiabetic, anticancer, anti-adipogenicity, hepato-protective effects and reduced atherosclerosis. (Sivalingam, Paramasivan, Weng, & padma, 2017) reported the bioactive compound neferine of reduced the dosage toxicity of the anticancer drug cisplatin in A549 cells under a combinatorial regimen. (Lin et al., 2019) reported the anti-obesity activity of phenolic compounds of lotus seeds by modulating the AMPK signalling pathway. (Wang et al., 2018) reported the antidiabetic properties of lotus seeds with genome-wide changes in mice. (Pan et al., 2021) showed that anti-hyperlipemia characterises lotus leave compounds through network pharmacology. Here we described the relationship between Nelumbinis semen (lotus seeds) compounds and biological targets of Atherosclerosis and Type 2 Diabetes Mellitus.
Materials and Methodology
Compound selection
The biologically active compounds of Nelumbinis semen were derived from (Arooj et al., 2021). A total of 62 bioactive compounds were taken. The compounds' chemical structures, represented as canonical SMILES and their PubChem Ids, were obtained from the PubChem database. (PubChem – accessed on 12th April 2023).
Target Identification
For target prediction, the canonical SMILES of the bioactive compounds were submitted to the Swiss TargetPrediction online tool (Swiss TargetPrediction – accessed on 12th April 2023) (Daina, Michielin, & Zoete, 2017). The targets were selected for further analysis after being filtered with a probability score greater than and equal to 0.1. The targets associated with Atherosclerosis and Diabetes Mellitus were retrieved from the Open Targets Platform (Open Targets Platform – accessed on 12th April 2023) (Ochoa et al., 2022) . The targets were filtered based on the overall associated score greater than and equal to 0.5. Target names and gene symbols were standardised using the UniProt database. Using Cytoscape_v3.9.1 software, the target network for the seed compounds was constructed and visualized in a degree-sorted circular layout. The shared targets were obtained from Venn Diagram, using Venny 2.1.0 (Venny 2.1.0 – accessed on 12th April 2023) (Oliveros, 2007-2015) .
Protein-Protein Interaction Network
The STRING 11.5 database (STRING 11.5 - accessed on 12th April 2023) was used to build the Protein-Protein Interaction network (Mering, Christian, Huynen, Jaeggi, & Schmidt, 2003). The network was constructed and examined using the minimum interaction threshold value of "high confidence >0.7". The network's edges' thickness reflected the strength of the data.
Gene and Pathway Enrichment Analysis
ShinyGo 0.77 (ShinyGo 0.77 - accessed on 3 April 2023) was utilised for the gene ontology and KEGG pathway analysis (Ge, Jung, & Yao, 2020). The top 20 biological processes and pathways were chosen based on the fold enrichment. The top 20 biological processes, cellular elements, and molecular functions of the genes were predicted and represented in the form of a lollipop plot (the colour and size of each lollipop represent the gene count and -log10FDR, respectively, and the X-axis and Y-axis show the fold enrichment and full names of the processes, respectively). The network was also produced based on the number of genes involved in the pathway and the proportion of overlapping genes (Kanehisa et al., 2021). The lollipop and hierarchical tree plots depicted the biological pathways.
Figure 1
Representation of predicted compound-target network in degree sorted circular layout. The blue colour represents lotus seed compounds, yellow colour represents the predicted targets and red colour represents the common genes for compound predicted targets and disease targets.
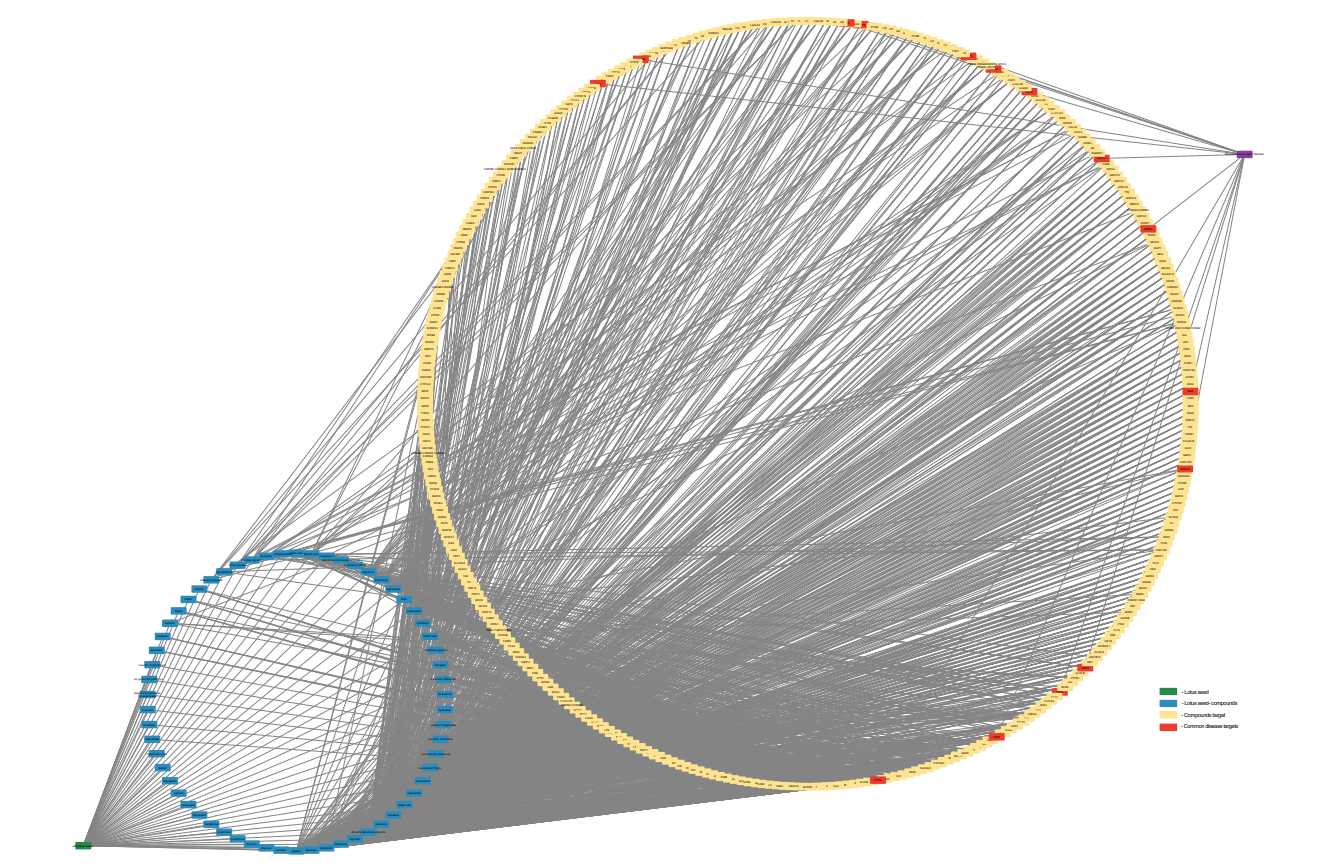
Figure 2
The Venn diagram shows the number of common genes between Nelumbinis semen (lotus seeds) and diseases (Atherosclerosis & Type 2 Diabetes). The total of 15 (10+3+2) common gene were found common.
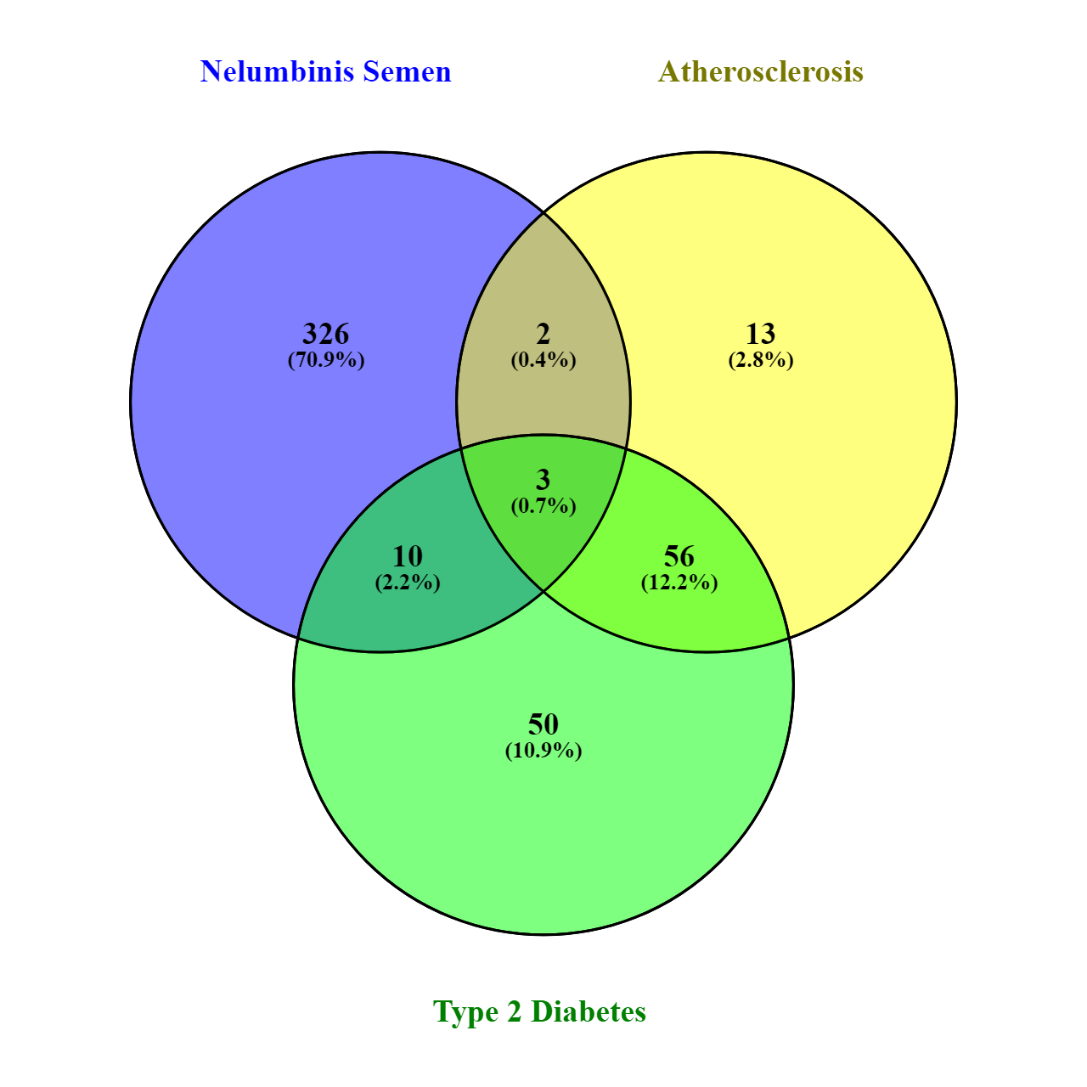
Table 1
The common gene between Nelumbinis semen and diseases (Atherosclerosis & Type 2 Diabetes)
Results and discussion
Target Identification
The lotus seed compounds’ targets were predicted using Swiss TargetPrediction, and the targets with a probability score greater than and equal to 0.1 were filtered (Figure 1). A total of 1978 targets were predicted for 50 compounds (the target list was attached to the supplementary information, Appendix A). The disease-associated targets for Atherosclerosis and Type 2 Diabetes Mellitus were retrieved and filtered with the overall associated score greater than and equal to 0.5, and a total of 75 and 120 targets were obtained, respectively. The common target genes were obtained from the Venn diagram, and 15 common target genes were obtained between Nelumbinis semen compounds and disease targets (Figure 2). The common targets are listed in Table 1. Even the Venn diagram witnessed the association between atherosclerosis and Type 2 mellitus with 59 common genes. A network was constructed and visualised in Degree sorted circular layout. Using the Cytohubba plugin, the nodes (compounds) with the highest degree and the nodes that are closely connected were found. Myricetin, Quercetin, Kampferol, Apigenin and Rhamnetin had the highest degree with a score of 101 and were closely associated with a score of 212.33.
Protein-Protein Interaction (PPI) Network
The STRING database created the protein-protein interaction network with a high confidence value >0.7 (Figure 3). The network of protein-protein interactions shows how proteins interact physically and functionally. The network had more significant interaction than the expected interaction. The interaction between HMGCR, NPC1L1 and PPARA showed that lotus seed compounds had a relationship with PPARα (Peroxisome proliferator-activated receptor alpha), which is ligand activated transcription factor highly expressed in the liver and plays a vital role in hepatic lipid metabolism (Kersten & Stienstra, 2017), HMG-Co Reductase and NPC1L1 which plays an important role in cholesterol homeostasis. The interaction between the Beta-adrenergic receptor 1 & 2 (ADRB1 and ADRB2) and the Insulin receptor (INSR) showed an association with diabetes mellitus because it maintains glucose homeostasis (Tosur et al., 2020).
Figure 5
Pathway enrichment (a) Lollipop plot of KEGG pathways (b) Hierarchical representation of pathway tree (c) Pathway network. In pathway network the size of the node represent the no.of genes involved in the pathway and the thickness of the edges represent the percentage of overlapping genes.
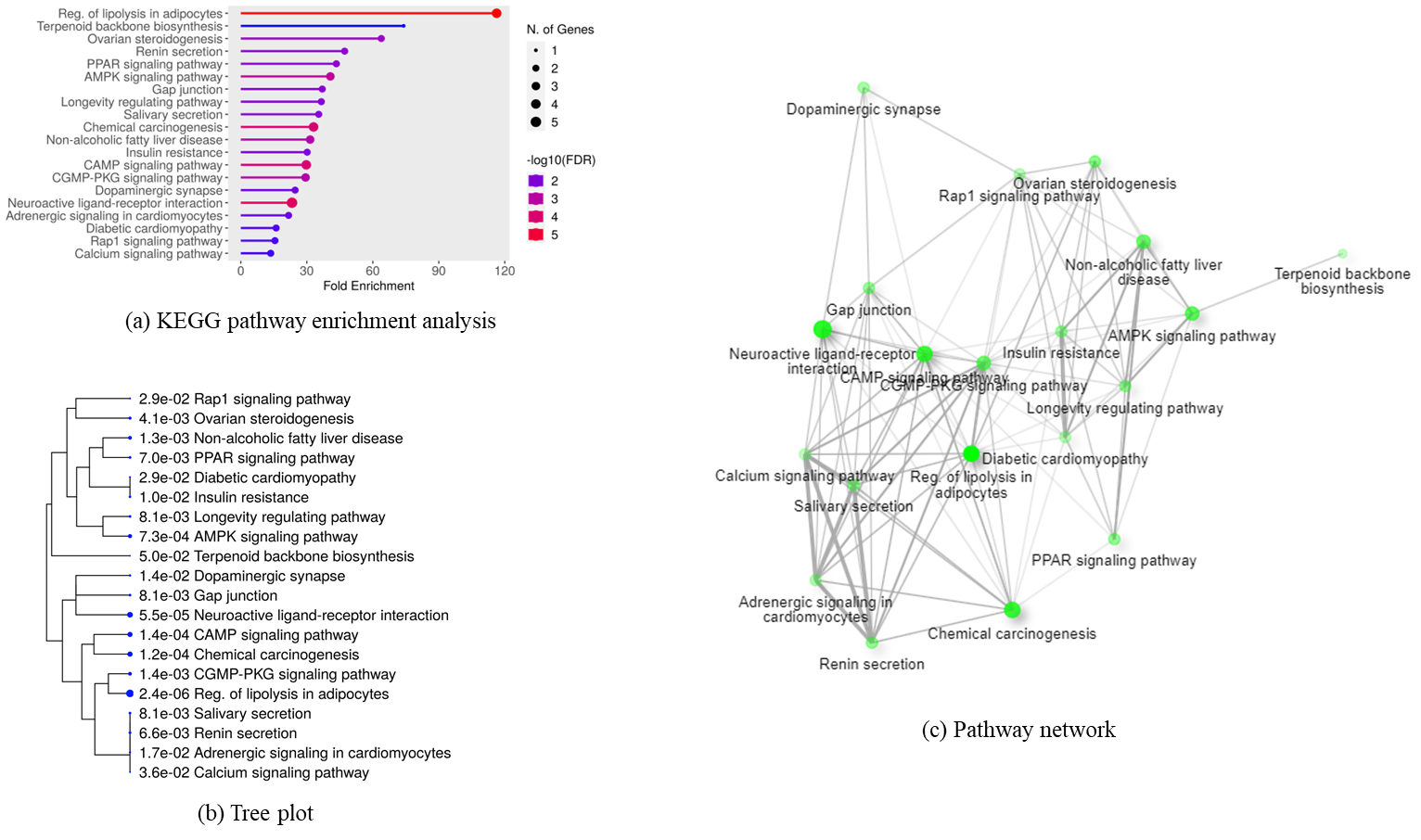
Table 2
The gene involved in the pathway and their associated compounds.
Gene and Pathway Enrichment Analysis
For enrichment analysis, the gene names of the common targets were imported into the online web application ShinyGo 0.77. With an FDR cut-off value of 0.05, the top 20 biological pathways and processes were obtained (Figure 6; Figure 5; Figure 4). The gene ontology of the common proteins for biological processes, cellular components, and molecular function was depicted as a lollipop plot, where the colour and size of each lollipop correspond to the gene count and -log10FDR, respectively, and the X-axis and Y-axis show the fold enrichment and full names of the processes, respectively. The compound’s predicted genes were found mainly in the lipolysis regulation pathway, terpenoid backbone biosynthesis, and non-alcoholic fatty liver diseases, highly associated with atherosclerosis and Diabetic cardiomyopathy, and insulin resistance highly associated with Type II Diabetes Mellitus. The compounds and their interacting genes are listed in Table 2. The compounds of Nelumbinis semen had a relationship with PPARα (Peroxisome proliferator-activated receptor alpha) and INSR (Insulin Receptor) in Diabetic cardiomyopathy. (Raalte et al., 2004) reviewed the promising aspects of peroxisome proliferator-activated receptor alpha as a potent target for atherosclerosis. In terpenoid biosynthesis, it is predicted that the compounds have biological activity against HMG-Co Reductase, which plays an important role in cholesterol biosynthesis (Patni, 2023), and the compound had an active role in the regulation of lipolysis in adipocytes. This shows the mechanism underlies the therapeutic effect of lotus seeds in Atherosclerosis. It is well known that the crucial role of the insulin receptor in diabetes (Bays, Bindlish, & Clayton, 2023). This study describes the relationship between lotus seeds and the therapeutic potential towards Type II diabetes Mellitus. Through this network pharmacology, the lotus seed compounds had a therapeutic effect in atherosclerosis and Type II diabetes with multiple targets like PPARα, INSR, HMGCR, NCp1L1, ADRB1 and ADRB2. These studies show that traditional medicines and medicinal compounds treat numerous diseases and targets.
Conclusion
The complete network pharmacology study on Nelumbinis semen (lotus seeds) phytochemical compounds elucidates the mechanism of therapeutic action in Atherosclerosis and Type 2 Diabetes Mellitus. The multiple compounds of lotus seed act on numerous targets for therapeutic action and are involved in various pathways associated with diseases. Traditional medicines and medicinal compounds cure the disease in a delayed process when to conventional drugs but cure entirely with no side effects. Further studies on the compounds of lotus seeds will illuminate new therapeutic formulations for patients with atherosclerosis and type 2 diabetes. The validation of the network pharmacology through molecular docking, gene expression microarray analysis, and western blotting gives a clear understanding of compounds' cumulative activity at the molecular level. Further in-vitro and in-vivo studies could help improve natural compounds' pharmacokinetics and pharmacodynamics properties.